AI-powered Process and Equipment Enhancement across the Industrial World
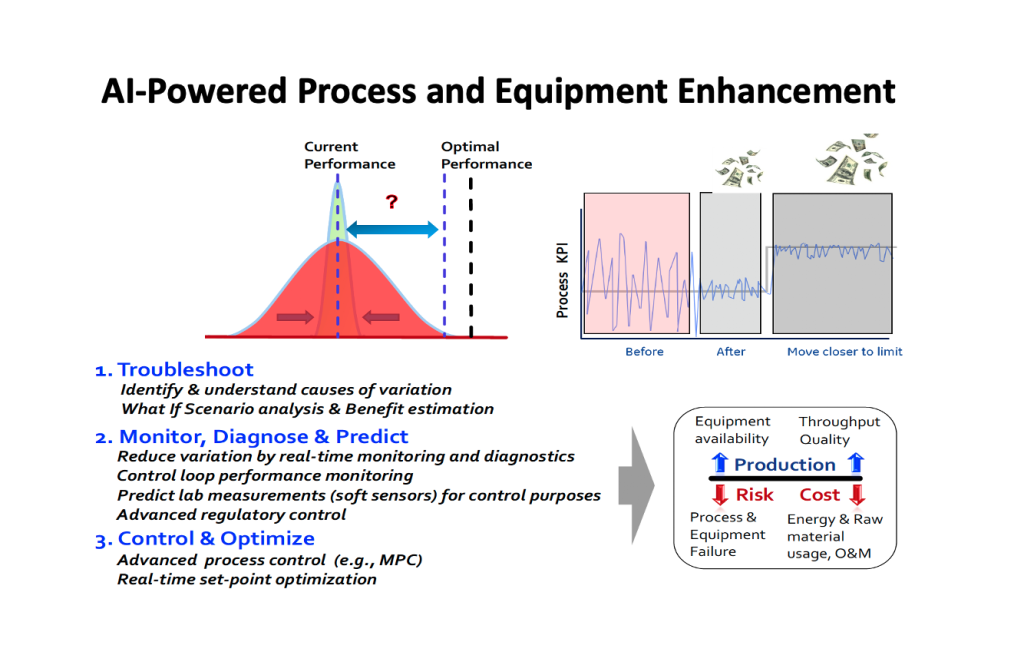
In follow-up to the following articles in the Democratizing AI Newsletter which focused on “AI as Key Exponential Technology in the Smart Technology Era“, “AI-driven Digital Transformation of the Business Enterprise“, and “AI Revolutionizing Personalized Engagement for Consumer Facing Businesses“, this article shares some text and audio extracts from Chapter 6, “”AI-powered Process and Equipment Enhancement across the Industrial World ” in the book Democratizing Artificial Intelligence to Benefit Everyone: Shaping a Better Future in the Smart Technology Era as it pertains to the following topics, which will also be discussed on 31 March 2022 at BiCstreet‘s “AI World Series” Live event (see more details at the bottom of the article):
- 21. Impact of AI on Utilities and the Energy Sector
- 22. Smart Technology Driven Resources and Manufacturing
- 23. AI Revolutionizing Agriculture
21. Impact of AI on Utilities and the Energy Sector
AI-powered Process and Equipment Enhancement across the Industrial World
As I have spent a substantial part of my AI career in applying AI to industrial world use cases, I share in this chapter also some of the specific AI use cases that I have been involved with via my AI companies, CSense Systems as well as Cortex Logic, along with many other illustrative real-world use case examples from around the globe. We continue our sense-making journey on the application front to specifically learn how the utilities, energy, resources, manufacturing, and agriculture sectors are getting powered by AI to enhance industrial processes and equipment related use cases with respect to monitoring, diagnostics, and predictive maintenance.
Impact of AI on Utilities and the Energy Sector
AI opens a wide range of exciting possibilities for utilities such as energy, gas, water, and waste management as well as the energy sector as a whole and can deliver real value to serious adopters and customers. The application of AI can not only assist the utility sector at every step of the value chain to provide consistent access to electricity and water that is essential for households and enterprise-level customers to help them live and do business, but also help to ease the transition to renewable energy, preventing power outages, reduce waste and learn more about end-users and their needs. It is also clear that there is a shift in the utilities and energy sector from a conservative, regulations-driven environment to a more advanced smart technology driven marketplace that relies on AI, data, and smart devices to better predict supply and demand, optimize infrastructure, balance the grid in real time, maximize yield, predict equipment failure, reduce downtime, deliver proactive condition-based maintenance, and improve customer-facing interactions and experience. From an electric utility’s perspective, apart from helping to make the smart grid smarter and reduce the need to add power plants, AI can also help to address issues linked to the growing complex network of power generation, transition and distribution stakeholders and assets, cost pressures, price deregulation, as well as the increasing volatility in energy supply with more renewable energy resources being deployed. A recent global survey revealed that there is general excitement and consensus about the potential of renewable energy and its sustainability benefits where the majority of people thought it is important to create a world fully powered by renewable energy and move away from a dependence on fossil fuels.[i] Although governments across the globe are supporting this drive with subsidies and tax credits, there have also recently been some cuts in governmental subsidies as we have seen in China.[ii] The Center for Climate and Energy Solutions reported that renewables was the fastest-growing energy source in the United States with a 100% increase from 2000 to 2018. It also made up 26.2% of global electricity generation in 2018, and is expected to rise to 45% by 2040, mainly driven by solar, wind, and hydropower.[iii] Given demand that also fluctuates heavily by time, geography, weather and events, the current energy grids with their legacy infrastructure struggle to optimally smooth out spikes and lose money with excess power. AI can also help to address these issues and help drive successful renewable projects, which in turn will encourage further investment into the use of renewables. There is also a massive shift towards increased electrification. Although efficiency gains will lead overall electric loads to decline, the Electric Power Research Institute (EPRI) projects that pursuing electrification would lead to cumulative load growth of between 24% and 52% with beneficiaries of increased electrification to include the utilities, electrical vehicles, heat pumps, warehouse equipment, and agriculture use cases.[iv] In a National Electrification Assessment that outlined the utility, customer and societal impacts of electricity providing up to 50% of final energy consumption by 2050, the EPRI also projected AI-driven positive outcomes such as an increased grid efficiency and flexibility, reduced energy consumption and consumer costs, better air quality leading to improved human health and much lower greenhouse gas emissions.[v] With accelerated adoption of electric vehicles, AI and load-level information can be used to benefit utilities as well as electric vehicle drivers. According to Bloomberg New Energy Finance (BNEF), sales of electric vehicles will increase from 1.7 million in 2020 to 8.5 million in 2025, 26 million in 2030 and 54 million in 2040.[vi] According to BIS Research, AI applications in the energy market is expected to reach $7.78 billion by 2024 with a projected CAGR of 22.49% from 2019 to 2024 driven by the growing concern for energy efficiency and battery storage systems, the demand for greater operational efficiency, and the significant increase in decentralized power generation.[vii] There are similar opportunities in the water utilities and waste water market where the application of AI and real-time smart water sensor measurement for decision intelligence, opex savings, process optimization and predictive maintenance is helping to drive this market to almost $750 billion in 2019 (according to a recent Frost & Sullivan report).[viii] There are also a range of AI use cases in the capital intensive oil and gas industry (where there has been traditionally many layers of redundancy as well as process and equipment over-engineering and inefficiencies to avoid failure) such as improving resource allocation, efficiency and reliability, real-time process and equipment monitoring, diagnostics and anomaly detection, predictive maintenance, forecasting, advanced process control and process performance enhancement. GS GIR estimated that a 1% reduction in the oil and gas industry’s capex, opex and inventory management can result in savings of about $140bn over a 10-year period.[ix]
Demand and supply prediction is one of the most impactful and critical use cases of AI for electrical utilities in the Smart Technology Era as they are working towards better information, efficiency, and safety to save costs, improve reliability and deliver a more flexible, agile, and robust smart grid that satisfies the growing energy demands from customers. Utilities use machine learning-based load forecasting algorithms to predict in real-time how much power must be generated to meet the short-, medium- or long-term demand of their customers, as well as optimize economic load dispatch. If load forecasts are inaccurate it can affect many stakeholders across the value chain, for example, power generation entities typically need a few days forecast to determine which power sources should be allocated for the next day, transmission grids have specific requirements to determine optimal resource allocation, and electricity retailers need to estimate the electricity demand for their energy price calculations. By modernizing electricity grids with embedded digital technology such as sensors, IoT devices, cloud-based data management platforms, high-speed communications networks to allow for improved interconnectedness, and other hardware and software innovations, the more data are being generated in digital format and can be leveraged for machine learning driven demand and supply prediction and other analytics purposes. A smart grid also helps to facilitate better information and operations technology convergence and ensure smarter, faster, and more efficient real-time solutions that support the integration of and access to distributed energy resources, better operational performance and adaptiveness to market needs, intelligent automation, predictive capabilities, communications, self-healing services, controls, and decision intelligence. Combining smart wires with machine learning based applications enable real-time power dispatching and the optimization of it to current grid load and to buildings’ asset portfolios. Real-time and more accurate forecasting makes it possible to integrate excess power and additional renewable energy into the smart grid, assess the reliability of those integrated generation assets, avoid or at least delay ramping up fossil-fuel-powered stations, and helps to automate demand-side responses.
Google DeepMind has provided a good supply prediction use case via their prediction of the energy output of the Google wind farms in the United States. Their machine learning based models were trained on historical wind turbine data and weather forecasts to predict wind power output 36 hours ahead of actual generation with an acceptable degree of accuracy (which is not easy to predict accurately due to the variable nature of wind) and made recommendations on how to make optimal hourly delivery commitments to the power grid 24 hours in advance, raising the “value” of their wind energy by approximately 20%.[x] The DeepMind team in England also started a collaboration with the UK National Grid a few years ago with the aim to predict supply and demand peaks and was hoping to reduce the UK’s national energy usage by 10%. Although ideas have been shared on how smart technology can improve grid efficiency and resilience, a solution has unfortunately not been implemented yet.[xi] With CSense Systems, we have also been involved in developing models to predict the residential electricity load on a substation level in South Africa with high accuracy using a range of inputs such as historical electricity usage, temperature parameters, time of day, day of the week, season, and weather. In another application, AI-based solutions have been developed to monitor and troubleshoot the health of transformers on a fleet level in the USA. The monitoring involves detecting overheating of hot spot development, solid insulation material contamination of oil which causes chemical degradation of the liquid, unbalanced and overloaded phases, restricted or blocked coolant flow, insulating oil quality, and inefficient transformer operation. The solution combines expert rules and fingerprint machine learning based models of ideal or good operation to monitor deviations from ideal behavior and to do real-time causal analysis.
Demand management can also be automated and made smarter with AI-based approaches. A case in point is provided by the Electric Reliability Council of Texas which operates their electric grid and manages the deregulated market for 75% of that state. It has been reported that in recent years there has been a demand response reduction of up to 2,000 MW as part of their AI-driven demand response program implemented for about 13,000 large customers and non-opt-in entities that can assess and quantify self-deployed load reductions in response to high dollar incentives.[xii] Another example of energy demand management is provided by NextEra Energy in the US and Canada that has been using AutoGrid platform and machine learning to help businesses reduce energy bills during times of high electric prices or peak energy demand.[xiii] In order to derive insights and predict electricity demand and power failures, the AI-driven solution makes use of petabytes of data from sensors, smart sensors, and other available relevant data. Open Energi in the UK has estimated that AI models that learn to control the electricity consumption of a portfolio of assets could be used to help unlock up to 6GW of demand-side flexibility without affecting end users.[xiv]
There are also significant business cases for the use of AI-based solutions to help reduce costs across operations (from power generation to transmission and distribution), improve electricity production yield, predict failures, optimize condition-based preventive maintenance, reduce energy waste, and prevent electricity theft. With respect to AI-driven yield optimization, power providers can maximize their generation efficiency with real-time monitoring and adjustments across their assets. As an example, GE Renewable Energy’s “Digital Wind Farm” can increase energy production by up to 20% to create $100 million in additional value over the lifetime of a 100-megawatt wind farm by using a machine learning based software solution that monitors and help optimize wind turbines’ yield based on their own past performance, real-time communication with other wind farms, the grid status, and changes in wind speed and direction.[xv] A wind turbine generates electricity by harnessing kinetic energy from the wind to turn the blades of the turbine and transform the rotational motion to electricity. One of the key problems with wind turbines is the blades of the turbine that are not always aligned to get the turbine to transfer the maximum amount of wind energy. If the blades are misaligned, there is less turbine rotation, which implies less electricity generated. In the ideal case, the amount of power generated (kilowatt) should be directly related to the speed of the wind. When at my previous AI company as well as at GE, I have been directly involved in developing machine learning based solutions for wind turbine troubleshooting, blade misalignment calculations, and real-time monitoring and intelligent alarming that provides real-time causal analysis for any anomalies or deviations.
(For more on this, read the paperback or e-book, or listen to the audiobook or podcast – see jacquesludik.com)
22. Smart Technology Driven Resources and Manufacturing
Smart Technology Driven Resources and Manufacturing
The resources industries such as minerals, metals, and mining as well as the manufacturing sector have seen a wide variety of impactful AI use cases over the years, many of them which I have experienced firsthand. Current economic realities mandate getting the most from existing assets and this is especially relevant in the capital-intensive industries such as mining and manufacturing were doing things the way they have always been done is no longer an option. Even minor improvements in production performance can make significant contributions to the bottom line, leading engineers to critically review the processes in their charge. But this can be a daunting and difficult task without the proper smart technology and tools because the processes that are most in need of improvement and that will yield the most profitable results are usually those that are too complex to optimize manually such as metal beneficiation. Over the last few decades, we have seen a significant increase in instrumentation and digitization across mining and manufacturing plants with more sensors and IoT devices along with industrial application layer applications such as programmable logic control systems, supervisory control and data acquisition systems, distributed control systems, manufacturing execution systems, maintenance systems, and enterprise resource planning systems. AI solutions capitalizing on all available structured and unstructured data can help to enhance every application across the industrial application spectrum. In the minerals, metals, and mining industries, we have especially seen AI being applied to the control and human computer interface layers for smarter control applications to enhance the standard PID control and improve support for operators, process engineers and production managers to better understand process and equipment behavior and help to troubleshoot and optimize their plant. To do this successfully, the first step is to thoroughly understand the process being addressed through modeling and simulation using historical data. The second is the implementation of AI-driven solutions that address the identified problem in real-time. Then comes the distribution of information in a form that is relevant to the people that must use it to make informed decisions – the information needs of management, process engineers, maintenance staff, automation personnel and operators, for example, are all different. Lastly, to be a long-term success, any process improvement programme must include sustained skills transfer, engineering assistance, training, and effective roll-out schedules as well as on-going assessment of the business value of the improvement. So, process performance enhancement involves more than just AI or software. It is all about the tools, the solutions, and the programme to bring it all together. Some relevant use cases for deploying AI solutions in the resources and mining sector include seismic data analysis, rapid process and equipment troubleshooting, real-time process and equipment monitoring, diagnostics and anomaly detection, predictive maintenance, advanced process control, and overall process performance enhancement. We have seen the same on the manufacturing side although the automotive and assembly sectors have shown higher AI adoption and digitization compared to for example consumer packaged goods and even building materials and construction which has been very low. Manufacturers are focused on improving product design yield and efficiency, optimize pricing, refine sales-leads prioritization, automate supplier assessment, anticipate parts requirements, reduce material delivery time, improve processes by the task, automate assembly lines, reduce errors, limit product rework, optimize flight planning and route and fleet allocation, enhance equipment maintenance engineering and training, and predict sales of maintenance services. AI can help to enhance each of these application areas. Some relevant use cases for deploying AI solutions in manufacturing sector includes real-time, batch, and discrete process monitoring and diagnostics; rapid process and manufacturing equipment troubleshooting; intelligent process control; optimizing supply chains and creating greater economies of scale; improving preventative maintenance; achieving better repair, maintenance, and overhaul performance with greater predictive accuracy to the component and part-level; increasing production yields by the optimization of the team, machine, supplier and customer requirements; providing more relevant data so finance, operations, and supply chain teams can better manage factory and demand-side constraints; knowing the right price to charge a given customer at the right time to get the most margin and closed sale; better machine senses can lead to safer workplaces; manufacturing-as-a-service with AI enabling subscription models for production service; the use of intelligent virtual assistants and advisors; and uber maintenance.
Apart from all these generic manufacturing-related use cases, AI in the automotive industry is also helping to pave the way for autonomous vehicles, where they are seen as a post-Uber disruption to public and good transportation. As we have seen with Tesla, Waymo, and others, machine learning based pattern recognition gives these vehicles the ability to identify their surroundings and to take actions in any given scenario at various levels of autonomy. This is done by feeding state-of-the-art AI systems with real-time data from sensors, cameras, GPS, lidar and cloud services to produce control signals that are used to operate the vehicle. Apart from helping to ensure proper and safe driving by for example enabling emergency control of the vehicle, active monitoring of blind spots and cross-traffic detection, it can also be used to monitor and learn driver and user behavior, predict and prescribe preferences based on user behavior, offer predictive and prescriptive maintenance of individual vehicles as data about the physical condition of the vehicle is stored in the cloud, and provide accurate data feeds for regulators with respect to traffic violations and insurance companies for driver risk assessment and to streamline the handling of claims. We also see AI-driven robotics use cases in manufacturing with industrial robots that can do tasks with high speed, accuracy, and endurance such as those in material handling, assembling, disassembling, welding, painting, dispensing, packing, labeling, inspecting, and testing. In the future, one might expect spectacular applications of AI and robotics in the resources and manufacturing industries that can include the use of dynamic highly mobile robots such as those being developed by Boston Dynamics in the form of Atlas, Handle, Pick and Spot.[i]
Given the continued expansion of the global economy and the rise of living standards, the Organization for Economic Co-operation and Development has projected in their Global Material Resources outlook that the world’s consumption of raw material is set to effectively double from approximately 90 Gigatons today to 167 Gigatons in 2060.[ii] Over the next few years the global smart mining market size is expected to reach $24 billion in 2027, from approximately $9.3 billion in 2019, which implies a compound annual growth rate of 13% from 2020 to 2027.[iii] Smart mining can be described as a process that uses data, information, technology such as AI and other state-of-the-art software solutions, and autonomy to gain better productivity, reduce operational costs, and enhance safety for a mine site. These smart technology-driven solutions also comprise remote-controlled robotic equipment for mineral and metal extraction to help decrease the danger for miners.
With the manufacturing sector accounting for almost 16% of the global GDP in 2018, it is clearly also a major part of the world economy and one of the key reasons governments across the globe is promoting the manufacturing sector, such as Make in India and Made in China.[iv] As an example, China wants to transform itself into a leading manufacturing power that moves up the manufacturing value chain by utilizing innovative manufacturing technologies or smart manufacturing, whereas India wants to reduce their dependence on exporting nations by producing goods in their own country, supported by significant foreign direct investment. However, with the outbreak of the COVID-19 pandemic, we have seen a significant decline in the global foreign direct investment. The United Nations Conference on Trade and Development has estimated that the COVID-19 could cause global foreign direct investment to shrink by 5%-15%, due to the downfall in the manufacturing sector along with factory shutdown with substantial negative impacts in the energy, chemicals, electronics, airlines, and automotive sectors and a shortage in raw materials.[v] Despite this knock on the manufacturing industry, the global application of AI in the manufacturing market is expected to still grow strongly with a compound annual growth rate of about 55% until 2025 when its size is projected to be approximately $15 billion.[vi] With radical shifts in demand and the increasing use of AI and other smart technology solutions, manufacturers are experiencing a growing disruption of the entire end-to-end value chain and forcing them to brainstorm new supply chain models, adopt new plant designs, and redefine their manufacturing footprints.
Process manufacturing in industries such as minerals, metals and mining, oil and gas, pulp and paper, refining, cement and glass, chemicals, electric power generation, food and beverage, life sciences, as well as water and wastewater typically utilizes physical, chemical, and compositional changes via continuous and/or batch processes to convert raw material or feedstock into a product. A subset of these industries such as life sciences, minerals, metals and mining, and fine chemicals include a mixture of continuous, sequential or batch and discrete processes that are often referred to as “hybrid” applications and industries. AI-driven process performance enhancement can be applied to continuous, batch or discrete processes as well as hybrids thereof. A continuous process is defined as the continuous flow of material through various processing equipment. Once a continuous process is operating in a steady state, the goal is to produce a consistent product at optimal yield levels. Continuous process manufacturing includes industries such as refining, chemical, petrochemical, and oil and gas applications and differs from discrete and batch processes manufacturing with respect to its non-stop operation, unscheduled downtime, high asset value, capacity utilization, and cost. In contrast, the nature of batch process manufacturing is typically shift-oriented with a low-volume/high-value focus, more agile, rapid response to market demand and high regulatory content. In general, a batch process is defined as a process that leads to the production of finite quantities of material by subjecting quantities of input materials to an ordered set of processing activities over a finite period of time using one or more pieces of equipment. Some examples of batch processes are biotech product manufacturing, pharmaceutical formulations, soap manufacturing, dairy processing, food processing and beverage processing. Discrete process manufacturing includes vertical markets such as industrial manufacturing, high technology, automotive, aerospace, defense, machinery, and electronics and produce items such as cars, airplanes, consumer electronics, computers and accessories, appliances, and other household items. A discrete process involves the production of things, where a part or a specific quantity of parts in a group moves from one workstation to another, gaining value at each location as work is performed. In a discrete process, each thing or part maintains its unique identity.
Process and equipment performance enhancement for the manufacturing and resources industries is about operationalizing end-to-end integrated AI-based solutions that delivers process and equipment troubleshooting, monitoring and optimization using all available structured and unstructured data. Rapid process or equipment troubleshooting involves identifying and understanding causes of variation in key performance indicators such as throughput, quality, and yield or key production, process or equipment related variables. To extract knowledge from process or equipment models, causal and sensitivity analysis is typically done on machine learning based AI models that are trained on data to be representative of specific process or equipment behavior. Further insights can be extracted by using these AI models in what-if-scenario analysis and benefit estimation simulations. These models can then also be used in real-time or batch fashion in monitoring and diagnostic solutions that feed off real-time or batch data. Other real-time applications include predicting lab measurements via software sensors for use in process control applications, AI-driven advanced process control and set-point optimization. Machine learning can also be applied in safety and security-related applications for identifying and tracking people in the work environment and detecting anomalous behavior. Other applications include smart sensors that use machine learning for pattern recognition on video and audio feeds which can complement the traditional sensors used to control processes and equipment.
Some of the most impactful use cases for process and equipment performance enhancement that I have personally been involved with via my AI companies CSense Systems as well as Cortex Logic more recently, include the stabilizing of the feed rate in a grinding process that led to a 5% increase in throughput for the mining company. In an equipment-related performance enhancement use case, the availability of haul trucks was increased from 70% to 85% using predictive analytics that focused on the engine, transmission, and braking characteristics of the haul trucks. In another equipment related use case, the Cortex AI Engine was used at a major diamond producer to increase throughput, yield, and reduce equipment risk through improved decision making and automated mining operations. To not have operational mining equipment available has a significant impact on revenue and operational costs. In this particular use case, a data mining solution helped to gain insights with respect to how geological features and diamond yield correlates during sampling. A machine learning based solution was then developed that, amongst others, maps hydrophone signals to geology in order to create signals that are used for improved predictive maintenance of key operational mining equipment and automated mining tools. Another AI use case for this diamond producer involved the use of machine learning based models to predict forward demand for diamonds and related categories across market, product category, and price category. This allows for optimization of diamond sales and the supply of rough stones to manufacturers and price it accordingly to meet demand.
(For more on this, read the paperback or e-book, or listen to the audiobook or podcast – see jacquesludik.com)
23. AI Revolutionizing Agriculture
AI Revolutionizing Agriculture
It is estimated that more than 1 billion people around the world work in agriculture with a contribution of about $2.4 trillion to the world economy.[i] So it is a significant foundation to the world economy. However, the agriculture industry across precision farming, livestock, aquaculture, and greenhouse farming is facing serious challenges from a growing world population with increased demand from food and related agricultural products. Assuming current growth rates, food production needs to increase by 70% to feed 9.1 billion people by 2050 where 70% will be urban and have higher income levels (the United Nations estimates 9.7 billion people).[ii] This can be addressed by using advanced farming techniques supported by smart technology driven farming solutions to increase the agriculture crop production as well the demand for protein-rich aqua food.[iii] As a result, the smart agriculture market has also seen significant growth from $13.8 billion in 2020 to $22 billion by 2025, at a compound aggregate growth rate of almost 10%.[iv] Goldman Sachs GIR has a similar estimate of approximately $20 billion by 2025 for the smart crop agriculture market and assumes a 25% value creation by AI and IoT for the crop agriculture market of $1.2 trillion by 2050.[v] They project that within just protein agriculture another $20 billion can be generated through the use of AI applications for precision breeding mechanisms as well as disease prevention and treatment. Apart from the pressure on the food supply system, modern farming techniques and growing income levels, this growth can also be attributed to increasingly instrumented smart agriculture and aquaculture farms as well as advanced livestock monitoring and disease detection to help increase farm yield by applying smart technologies such as AI and IoT along with global positioning systems, drones, sensors, radio-frequency identification, and light emitting diode grow lights. With this type of instrumentation huge sets of soil, satellite and aerial imagery, weather, temperature, precipitation, wind speed, solar radiation, video, and audio data can be assembled for monitoring, data mining and predictive analytics purposes. It is also clear that yield monitoring applications using precision farming technologies can provide farmers with the information, predictions and insights about soil properties, fertilizers, and weather conditions. As an example, GS GIS estimates that corn yields in the USA can be improved by 70% by 2050 by using AI-driven technologies such as precision fertilizer and compaction reduction.[vi] They also project that from a global crop agriculture value perspective, potential increases in global crop yields from smart technology based solutions could generate more than $800 billion in increased value by 2050 through precision irrigation, precision planting, precision fertilizer, precision spraying, compaction reduction, and moisture sensing. Machine learning solutions can also help to decrease irrigation and fertilizer costs, reduce labor costs, improve the quality of the agriculture products, detect potential crop or livestock diseases, increase the productivity of human workers as well as land and equipment use, and enhance decision-making with respect to irrigation, fertilization, planting times, and livestock care. Some specific use cases for deploying AI solutions in the agriculture sector includes crop harvesting and soil monitoring, predictive analytics of environmental impact on crop yields, determining time sowing, weed control, diagnosing soil defects, automated irrigation systems, crop health monitoring, saving plants, help IoT achieve its maximum potential in precision agriculture, image recognition and insights, intelligent virtual assistants and advisors for farmers, agricultural robots, drones, and driverless or smart tractors. These smart autonomous tractors can for example be trained or programmed to independently make decisions on speed of operation, detecting their ploughing position and avoid obstacles in the farm fields while performing various tasks.
Deforestation and degradation of land, in particular soil quality, remains a key global issue for this century due to its negative impact on the food security, agriculture and economic productivity, quality of life, and the environment. The cost for erosion from agriculture in the USA alone is already about $44 billion per annum. Peat.ai’s Plantix solution makes use of machine learning driven image recognition to diagnose infected crops and offers treatments for any disease, pest or nutrient deficiency issues.[vii] Another AI-driven soil defect analysis solution to help optimize healthy crop production is the one from Trace Genomics that utilizes soil science, genomics and machine learning to measure the bacteria and fungi in soil that cause disease and cycle nutrients and combine those measurements with soil chemical characteristics.[viii] Agricultural robots can help to increase production yields at higher volume and a faster pace compared to human workers by for example using drones, robotic arms, and autonomous tractors to automate some common repetitive tasks such as mowing, harvesting, picking, sorting, packing, pruning, thinning, spraying, seeding, controlling weed, planting seeds, and milking and washing livestock. Other benefits of these smart technology solutions are lowering production costs, decreasing the need for manual labor, and producing higher quality of fresh produce. According to estimation by the Weed Science Society of America the impact of uncontrolled weeds on soybean and corn crops in the USA and Canada has led to the annual losses to farmers of approximately $43 billion.[ix] Ecorobotix’s solar powered weeding robotic platform called AVA provides high precision targeted application of herbicide for ecological and economical weeding of row crops, intercropping cultures and meadows, whereas their fully autonomous solar-powered robot platform ARA is used for scouting and phenotyping applications and can move according to a predefined real-time kinematic global positioning system trajectory in autonomous fashion whilst minimizing soil compaction.[x] Blue River Technology which is now part of John Deere, has a robot called See & Spray which uses AI-driven machine vision to provide precision spraying of weeds on cotton plants, which also helps prevent herbicide resistance.[xi] Naio Technologies also has a range of robots that can weed, hoe and assist during harvesting of vegetable crops and vineyards.[xii] Energid provides a robot that can pick citrus fruit every 2-3 seconds at comparable cost to human labor, whereas Harvest CROO Robotics’ robot helps strawberry farmers pick and pack their crops at a rate of 8 acres a day and matching the equivalent of 30 human workers.[xiii]
Drones are also starting to play a significant role in monitoring large crop areas. Aerobotics in South Africa is for example using drone imagery and AI to get insights and identify problematic trees as well as assist with the management of orchards, pests, diseases and provide yield estimations.[xiv] Vineview is another leading provider that leverages aerial technology and AI to monitor crop health with their use of aerial-based sensors, machine learning and other advanced algorithms to help grape growers make responsive and informed vineyard management decisions.[xv] Vineview’s solutions involve achieving vine balance, optimizing harvest scheduling, eradicating grapevine disease in vineyards, and improving irrigation and conservation of water. Agribotix’s drone-enabled FarmLens data analytics platform, which is now part of AgEagle’s service offerings, provide the agricultural supply chain with AI-driven advanced analytic capabilities to identify areas where they can build soil health and reduce water or chemical usage.[xvi] Vision Robotics has a range of agricultural mechanization solutions that includes a lettuce thinner, weeder, an autonomous intelligent grapevine pruner, and crop load estimation for apples on a tree, strawberries on a plant and branches on a vines.[xvii] One of the USA-based companies that specializes in providing machine learning driven weather intelligence is aWhere which provides in-time weather, maps for economic resilience, weather insights and predictions, crop sustainability analysis, pest and disease modeling, fertilizer timing recommendations, and other related digital agriculture solutions.[xviii]
(For more on this, read the paperback or e-book, or listen to the audiobook or podcast – see jacquesludik.com)
BicStreet
This Democratizing AI Newsletter coincides with the launch of BiCstreet‘s “AI World Series” Live event, which kicked off both virtually and in-person (limited) from 10 March 2022, where Democratizing AI to Benefit Everyone is discussed in more detail over a 10-week AI World Series programme. The event is an excellent opportunity for companies, startups, governments, organisations and white collar professionals all over the world, to understand why Artificial Intelligence is critical towards strategic growth for any department or genre. (To book your tickets to this global event click the link below and enter this Coupon Code to get 5% Discount: Enter Coupon Code: JACQUES001 (Purchase Tickets here: https://www.BiCflix.com; See the 10 Weekly Program here: https://www.BiCstreet.com)).
Background LinkedIn Articles on Democratizing Artificial Intelligence
AI as Key Exponential Technology in the Smart Technology Era
AI-driven Digital Transformation of the Business Enterprise
AI Revolutionizing Personalized Engagement for Consumer Facing Businesses
Audiobook “Democratizing AI to Benefit Everyone” available world-wide
Launching the Jacques Ludik Podcast and YouTube Channels – Democratizing AI To Benefit Everyone
The audio book version of “Democratizing Artificial Intelligence to Benefit Everyone” is also available via major audio book market place world-wide. See details on my website as well as below. You can also listen to audio content of this book on the Jacques Ludik YouTube Channel or Jacques Ludik Podcasts. This release is in follow-up to the e-book (Kindle) and paperback version of the book that was released earlier this year on Amazon with some further updates recently.
For some background, see also the following introductory articles Democratizing AI to Benefit Everyone and AI Perspectives, Democratizing Human-centric AI in Africa, and Acknowledgements – Democratizing AI to Benefit Everyone (as well as United Nations & Democratizing AI to Benefit Everyone; World Economic Forum and Democratizing AI to Benefit Everyone; OECD and Democratizing AI to Benefit Everyone; AI for Good and Democratizing AI to Benefit Everyone).
For further details, see jacquesludik.com.
References
Impact of AI on Utilities and the Energy Sector
[i] https://www.vox.com/energy-and-environment/2017/11/20/16678350/global-support-clean-energy & https://www.smart-energy.com/renewable-energy/71-of-uk-citizens-say-renewables-need-more-government-support/
[ii] https://www.caixinglobal.com/2020-03-11/china-to-slash-subsidies-for-renewable-energy-amid-drive-to-cut-state-support-101527138.html & https://www.power-technology.com/features/renewable-subsidies-balancing-economy-and-the-environment-in-the-netherlands-and-beyond/https://www.power-technology.com/features/renewable-subsidies-balancing-economy-and-the-environment-in-the-netherlands-and-beyond/
[iii] https://www.c2es.org/content/renewable-energy/#:~:text=Renewable%20energy%20is%20the%20fastest,wind%20power%20(6.6%20percent).
[iv] https://www.utilitydive.com/news/ai-in-the-utility-industry/543876/
[v] http://ipu.msu.edu/wp-content/uploads/2018/04/EPRI-Electrification-Report-2018.pdf
[vi] https://about.bnef.com/electric-vehicle-outlook/
[vii] https://bisresearch.com/industry-report/artificial-intelligence-energy-market.html
[viii] https://go.frost.com/EI_PR_EE_KCekani_ME8E_WasteWater_Jul19
[ix] https://www.gspublishing.com/content/research/en/reports/2019/09/04/a0d36f41-b16a-4788-9ac5-68ddbc941fa9.pdf
[x] https://www.forbes.com/sites/samshead/2019/02/27/deepmind-and-google-train-ai-to-predict-energy-output-of-wind-farms/#7d6c81685e9e
[xi] https://www.forbes.com/sites/samshead/2019/03/06/google-deepminds-talks-with-national-grid-are-over/
[xii] https://utilityanalytics.com/2019/04/utilities-machine-learning-artificial-intelligence/
[xiii] https://www.auto-grid.com/news/nextera-energy-services-teams-up-with-autogrid-to-offer-new-demand-response-programs-in-pjm/
[xiv] http://powerresponsive.com/ai-shaping-future-energy/
[xv] https://www.ge.com/renewableenergy/sites/default/files/related_documents/GE_Digital_Transformation.PDF
Smart Technology Driven Resources and Manufacturing
[i] https://www.bostondynamics.com/
[ii] https://www.oecd.org/environment/waste/highlights-global-material-resources-outlook-to-2060.pdf
[iii] https://www.researchandmarkets.com/reports/5031470/smart-mining-market-by-type-and-category-global?utm_source=CI&utm_medium=PressRelease&utm_code=pmtjrk&utm_campaign=1414136+-+Insights+on+the+Smart+Mining+Global+Market+to+2027+-+Opportunity+Analysis+and+Industry+Forecast&utm_exec=jamu273prd
[iv] https://www.researchandmarkets.com/reports/5013542/impact-of-covid-19-on-the-global-manufacturing?utm_source=dynamic&utm_medium=BW&utm_code=q4bjsh&utm_campaign=1378625+-+Global+Manufacturing+Industry+Report+2020%3a+Deviations+in+Growth+Rates+due+to+the+COVID-19+Pandemic&utm_exec=joca220bwd
[v] https://www.researchandmarkets.com/reports/5013542/impact-of-covid-19-on-the-global-manufacturing?utm_source=dynamic&utm_medium=BW&utm_code=q4bjsh&utm_campaign=1378625+-+Global+Manufacturing+Industry+Report+2020%3a+Deviations+in+Growth+Rates+due+to+the+COVID-19+Pandemic&utm_exec=joca220bwd
[vi] https://www.alliedmarketresearch.com/press-release/artificial-intelligence-in-manufacturing-market.html
AI Revolutionizing Agriculture
[i] https://croplife.org/news/agriculture-a-2-4-trillion-industry-worth-protecting/
[ii] http://www.fao.org/fileadmin/templates/wsfs/docs/expert_paper/How_to_Feed_the_World_in_2050.pdf
[iii] https://www.sciencedirect.com/science/article/pii/S2590332219301320
[iv] https://www.marketsandmarkets.com/PressReleases/smart-agriculture.asp
[v] https://www.gspublishing.com/content/research/en/reports/2019/09/04/a0d36f41-b16a-4788-9ac5-68ddbc941fa9.pdf
[vi] https://www.gspublishing.com/content/research/en/reports/2019/09/04/a0d36f41-b16a-4788-9ac5-68ddbc941fa9.pdf
[viii] https://tracegenomics.com/
[ix] http://wssa.net/2016/05/wssa-calculates-billions-in-potential-economic-losses-from-uncontrolled-weeds/
[x] https://www.ecorobotix.com/en/
[xi] http://www.bluerivertechnology.com/
[xii] https://www.naio-technologies.com/en/
[xiii] https://www.energid.com/industries/agricultural-robotics ; https://harvestcroo.com/about/#why-harvest-croo
[xiv] https://www.aerobotics.com/
[xv] https://www.vineview.com/
[xvi] https://www.ageagle.com/drones